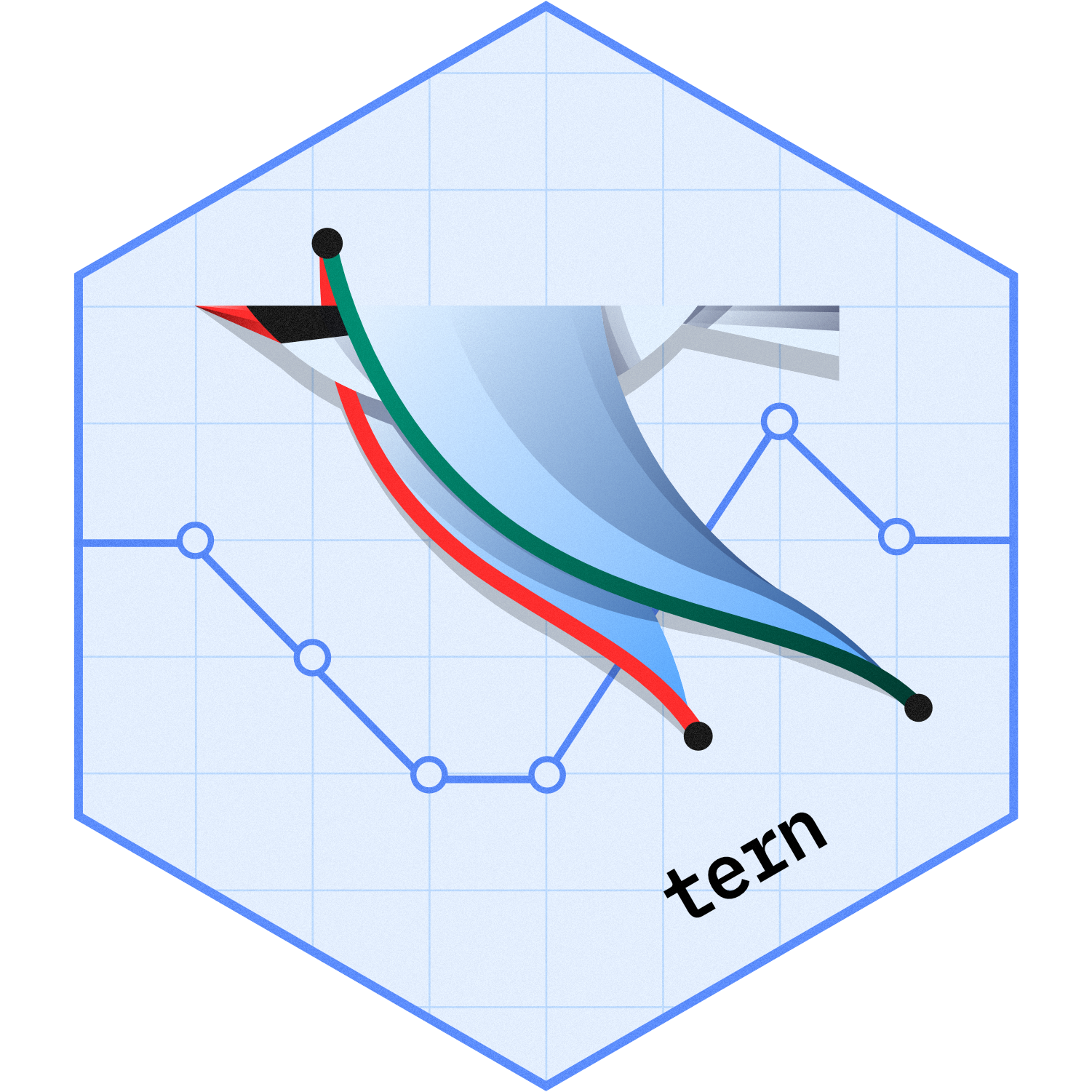
Helper functions for calculating proportion confidence intervals
Source:R/estimate_proportion.R
h_proportions.Rd
Functions to calculate different proportion confidence intervals for use in estimate_proportion()
.
Usage
prop_wilson(rsp, conf_level, correct = FALSE)
prop_strat_wilson(
rsp,
strata,
weights = NULL,
conf_level = 0.95,
max_iterations = NULL,
correct = FALSE
)
prop_clopper_pearson(rsp, conf_level)
prop_wald(rsp, conf_level, correct = FALSE)
prop_agresti_coull(rsp, conf_level)
prop_jeffreys(rsp, conf_level)
Arguments
- rsp
(
logical
)
vector indicating whether each subject is a responder or not.- conf_level
(
proportion
)
confidence level of the interval.- correct
(
flag
)
whether to apply continuity correction.- strata
(
factor
)
variable with one level per stratum and same length asrsp
.- weights
(
numeric
orNULL
)
weights for each level of the strata. IfNULL
, they are estimated using the iterative algorithm proposed in Yan and Su (2010) that minimizes the weighted squared length of the confidence interval.- max_iterations
(
count
)
maximum number of iterations for the iterative procedure used to find estimates of optimal weights.
Functions
prop_wilson()
: Calculates the Wilson interval by callingstats::prop.test()
. Also referred to as Wilson score interval.prop_strat_wilson()
: Calculates the stratified Wilson confidence interval for unequal proportions as described in Yan and Su (2010)prop_clopper_pearson()
: Calculates the Clopper-Pearson interval by callingstats::binom.test()
. Also referred to as theexact
method.prop_wald()
: Calculates the Wald interval by following the usual textbook definition for a single proportion confidence interval using the normal approximation.prop_agresti_coull()
: Calculates the Agresti-Coull interval. Constructed (for 95% CI) by adding two successes and two failures to the data and then using the Wald formula to construct a CI.prop_jeffreys()
: Calculates the Jeffreys interval, an equal-tailed interval based on the non-informative Jeffreys prior for a binomial proportion.
References
Yan X, Su XG (2010). “Stratified Wilson and Newcombe Confidence Intervals for Multiple Binomial Proportions.” Stat. Biopharm. Res., 2(3), 329–335.
See also
estimate_proportion, descriptive function d_proportion()
,
and helper functions strata_normal_quantile()
and update_weights_strat_wilson()
.
Examples
rsp <- c(
TRUE, TRUE, TRUE, TRUE, TRUE,
FALSE, FALSE, FALSE, FALSE, FALSE
)
prop_wilson(rsp, conf_level = 0.9)
#> [1] 0.2692718 0.7307282
# Stratified Wilson confidence interval with unequal probabilities
set.seed(1)
rsp <- sample(c(TRUE, FALSE), 100, TRUE)
strata_data <- data.frame(
"f1" = sample(c("a", "b"), 100, TRUE),
"f2" = sample(c("x", "y", "z"), 100, TRUE),
stringsAsFactors = TRUE
)
strata <- interaction(strata_data)
n_strata <- ncol(table(rsp, strata)) # Number of strata
prop_strat_wilson(
rsp = rsp, strata = strata,
conf_level = 0.90
)
#> $conf_int
#> lower upper
#> 0.4072891 0.5647887
#>
#> $weights
#> a.x b.x a.y b.y a.z b.z
#> 0.2074199 0.1776464 0.1915610 0.1604678 0.1351096 0.1277952
#>
# Not automatic setting of weights
prop_strat_wilson(
rsp = rsp, strata = strata,
weights = rep(1 / n_strata, n_strata),
conf_level = 0.90
)
#> $conf_int
#> lower upper
#> 0.4190436 0.5789733
#>
prop_clopper_pearson(rsp, conf_level = .95)
#> [1] 0.3886442 0.5919637
prop_wald(rsp, conf_level = 0.95)
#> [1] 0.3920214 0.5879786
prop_wald(rsp, conf_level = 0.95, correct = TRUE)
#> [1] 0.3870214 0.5929786
prop_agresti_coull(rsp, conf_level = 0.95)
#> [1] 0.3942193 0.5865206
prop_jeffreys(rsp, conf_level = 0.95)
#> [1] 0.3934779 0.5870917