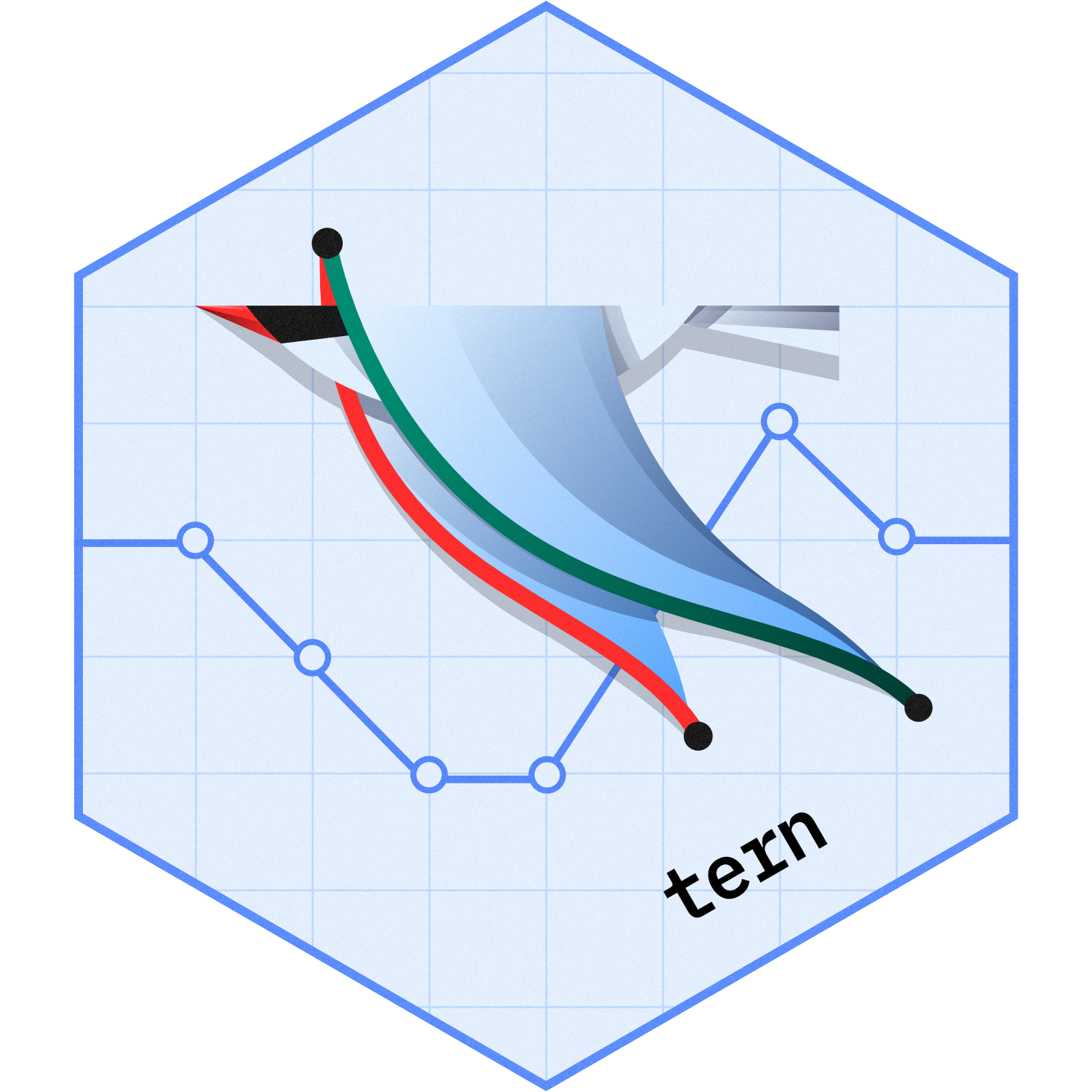
Tabulate survival duration by subgroup
Source:R/survival_duration_subgroups.R
survival_duration_subgroups.Rd
Usage
tabulate_survival_subgroups(
lyt,
df,
vars = c("n_tot_events", "n_events", "median", "hr", "ci"),
groups_lists = list(),
label_all = lifecycle::deprecated(),
time_unit = NULL,
na_str = default_na_str()
)
a_survival_subgroups(
.formats = list(n = "xx", n_events = "xx", n_tot_events = "xx", median = "xx.x", n_tot
= "xx", hr = list(format_extreme_values(2L)), ci =
list(format_extreme_values_ci(2L)), pval = "x.xxxx | (<0.0001)"),
na_str = default_na_str()
)
Arguments
- lyt
(
PreDataTableLayouts
)
layout that analyses will be added to.- df
(
list
)
list of data frames containing all analysis variables. List should be created usingextract_survival_subgroups()
.- vars
-
(
character
)
the names of statistics to be reported among:n_tot_events
: Total number of events per group.n_events
: Number of events per group.n_tot
: Total number of observations per group.n
: Number of observations per group.median
: Median survival time.hr
: Hazard ratio.ci
: Confidence interval of hazard ratio.pval
: p-value of the effect. Note, one of the statisticsn_tot
andn_tot_events
, as well as bothhr
andci
are required.
- groups_lists
(named
list
oflist
)
optionally contains for eachsubgroups
variable a list, which specifies the new group levels via the names and the levels that belong to it in the character vectors that are elements of the list.- label_all
please assign thelabel_all
parameter within theextract_survival_subgroups()
function when creatingdf
.- time_unit
(
string
)
label with unit of median survival time. DefaultNULL
skips displaying unit.- na_str
(
string
)
string used to replace allNA
or empty values in the output.- .formats
(named
character
orlist
)
formats for the statistics. See Details inanalyze_vars
for more information on the"auto"
setting.
Value
An rtables
table summarizing survival by subgroup.
a_survival_subgroups()
returns the corresponding list with formattedrtables::CellValue()
.
Details
These functions create a layout starting from a data frame which contains the required statistics. Tables typically used as part of forest plot.
Functions
tabulate_survival_subgroups()
: Table-creating function which creates a table summarizing survival by subgroup. This function is a wrapper forrtables::analyze_colvars()
andrtables::summarize_row_groups()
.a_survival_subgroups()
: Formatted analysis function which is used asafun
intabulate_survival_subgroups()
.
Examples
library(dplyr)
adtte <- tern_ex_adtte
# Save variable labels before data processing steps.
adtte_labels <- formatters::var_labels(adtte)
adtte_f <- adtte %>%
filter(
PARAMCD == "OS",
ARM %in% c("B: Placebo", "A: Drug X"),
SEX %in% c("M", "F")
) %>%
mutate(
# Reorder levels of ARM to display reference arm before treatment arm.
ARM = droplevels(forcats::fct_relevel(ARM, "B: Placebo")),
SEX = droplevels(SEX),
AVALU = as.character(AVALU),
is_event = CNSR == 0
)
labels <- c(
"ARM" = adtte_labels[["ARM"]],
"SEX" = adtte_labels[["SEX"]],
"AVALU" = adtte_labels[["AVALU"]],
"is_event" = "Event Flag"
)
formatters::var_labels(adtte_f)[names(labels)] <- labels
df <- extract_survival_subgroups(
variables = list(
tte = "AVAL",
is_event = "is_event",
arm = "ARM", subgroups = c("SEX", "BMRKR2")
),
label_all = "Total Patients",
data = adtte_f
)
df
#> $survtime
#> arm n n_events median subgroup var
#> 1 B: Placebo 73 57 727.8043 Total Patients ALL
#> 2 A: Drug X 69 44 974.6402 Total Patients ALL
#> 3 B: Placebo 40 31 599.1772 F SEX
#> 4 A: Drug X 38 24 1016.2982 F SEX
#> 5 B: Placebo 33 26 888.4916 M SEX
#> 6 A: Drug X 31 20 974.6402 M SEX
#> 7 B: Placebo 24 21 735.4722 LOW BMRKR2
#> 8 A: Drug X 26 15 974.6402 LOW BMRKR2
#> 9 B: Placebo 23 14 731.8352 MEDIUM BMRKR2
#> 10 A: Drug X 26 17 964.2197 MEDIUM BMRKR2
#> 11 B: Placebo 26 22 654.8245 HIGH BMRKR2
#> 12 A: Drug X 17 12 1016.2982 HIGH BMRKR2
#> var_label row_type
#> 1 Total Patients content
#> 2 Total Patients content
#> 3 Sex analysis
#> 4 Sex analysis
#> 5 Sex analysis
#> 6 Sex analysis
#> 7 Continuous Level Biomarker 2 analysis
#> 8 Continuous Level Biomarker 2 analysis
#> 9 Continuous Level Biomarker 2 analysis
#> 10 Continuous Level Biomarker 2 analysis
#> 11 Continuous Level Biomarker 2 analysis
#> 12 Continuous Level Biomarker 2 analysis
#>
#> $hr
#> arm n_tot n_tot_events hr lcl ucl conf_level pval
#> 1 142 101 0.7108557 0.4779138 1.0573368 0.95 0.09049511
#> 2 78 55 0.5595391 0.3246658 0.9643271 0.95 0.03411759
#> 3 64 46 0.9102874 0.5032732 1.6464678 0.95 0.75582028
#> 4 50 36 0.7617717 0.3854349 1.5055617 0.95 0.43236030
#> 5 49 31 0.7651261 0.3641277 1.6077269 0.95 0.47860004
#> 6 43 34 0.6662356 0.3257413 1.3626456 0.95 0.26285846
#> pval_label subgroup var var_label
#> 1 p-value (log-rank) Total Patients ALL Total Patients
#> 2 p-value (log-rank) F SEX Sex
#> 3 p-value (log-rank) M SEX Sex
#> 4 p-value (log-rank) LOW BMRKR2 Continuous Level Biomarker 2
#> 5 p-value (log-rank) MEDIUM BMRKR2 Continuous Level Biomarker 2
#> 6 p-value (log-rank) HIGH BMRKR2 Continuous Level Biomarker 2
#> row_type
#> 1 content
#> 2 analysis
#> 3 analysis
#> 4 analysis
#> 5 analysis
#> 6 analysis
#>
df_grouped <- extract_survival_subgroups(
variables = list(
tte = "AVAL",
is_event = "is_event",
arm = "ARM", subgroups = c("SEX", "BMRKR2")
),
data = adtte_f,
groups_lists = list(
BMRKR2 = list(
"low" = "LOW",
"low/medium" = c("LOW", "MEDIUM"),
"low/medium/high" = c("LOW", "MEDIUM", "HIGH")
)
)
)
df_grouped
#> $survtime
#> arm n n_events median subgroup var
#> 1 B: Placebo 73 57 727.8043 All Patients ALL
#> 2 A: Drug X 69 44 974.6402 All Patients ALL
#> 3 B: Placebo 40 31 599.1772 F SEX
#> 4 A: Drug X 38 24 1016.2982 F SEX
#> 5 B: Placebo 33 26 888.4916 M SEX
#> 6 A: Drug X 31 20 974.6402 M SEX
#> 7 B: Placebo 24 21 735.4722 low BMRKR2
#> 8 A: Drug X 26 15 974.6402 low BMRKR2
#> 9 B: Placebo 47 35 735.4722 low/medium BMRKR2
#> 10 A: Drug X 52 32 964.2197 low/medium BMRKR2
#> 11 B: Placebo 73 57 727.8043 low/medium/high BMRKR2
#> 12 A: Drug X 69 44 974.6402 low/medium/high BMRKR2
#> var_label row_type
#> 1 All Patients content
#> 2 All Patients content
#> 3 Sex analysis
#> 4 Sex analysis
#> 5 Sex analysis
#> 6 Sex analysis
#> 7 Continuous Level Biomarker 2 analysis
#> 8 Continuous Level Biomarker 2 analysis
#> 9 Continuous Level Biomarker 2 analysis
#> 10 Continuous Level Biomarker 2 analysis
#> 11 Continuous Level Biomarker 2 analysis
#> 12 Continuous Level Biomarker 2 analysis
#>
#> $hr
#> arm n_tot n_tot_events hr lcl ucl conf_level pval
#> 1 142 101 0.7108557 0.4779138 1.0573368 0.95 0.09049511
#> 2 78 55 0.5595391 0.3246658 0.9643271 0.95 0.03411759
#> 3 64 46 0.9102874 0.5032732 1.6464678 0.95 0.75582028
#> 4 50 36 0.7617717 0.3854349 1.5055617 0.95 0.43236030
#> 5 99 67 0.7472958 0.4600419 1.2139136 0.95 0.23764314
#> 6 142 101 0.7108557 0.4779138 1.0573368 0.95 0.09049511
#> pval_label subgroup var var_label
#> 1 p-value (log-rank) All Patients ALL All Patients
#> 2 p-value (log-rank) F SEX Sex
#> 3 p-value (log-rank) M SEX Sex
#> 4 p-value (log-rank) low BMRKR2 Continuous Level Biomarker 2
#> 5 p-value (log-rank) low/medium BMRKR2 Continuous Level Biomarker 2
#> 6 p-value (log-rank) low/medium/high BMRKR2 Continuous Level Biomarker 2
#> row_type
#> 1 content
#> 2 analysis
#> 3 analysis
#> 4 analysis
#> 5 analysis
#> 6 analysis
#>
## Table with default columns.
basic_table() %>%
tabulate_survival_subgroups(df, time_unit = adtte_f$AVALU[1])
#> B: Placebo A: Drug X
#> Baseline Risk Factors Total Events Events Median (DAYS) Events Median (DAYS) Hazard Ratio 95% Wald CI
#> ———————————————————————————————————————————————————————————————————————————————————————————————————————————————————————————
#> Total Patients 101 57 727.8 44 974.6 0.71 (0.48, 1.06)
#> Sex
#> F 55 31 599.2 24 1016.3 0.56 (0.32, 0.96)
#> M 46 26 888.5 20 974.6 0.91 (0.50, 1.65)
#> Continuous Level Biomarker 2
#> LOW 36 21 735.5 15 974.6 0.76 (0.39, 1.51)
#> MEDIUM 31 14 731.8 17 964.2 0.77 (0.36, 1.61)
#> HIGH 34 22 654.8 12 1016.3 0.67 (0.33, 1.36)
## Table with a manually chosen set of columns: adding "pval".
basic_table() %>%
tabulate_survival_subgroups(
df = df,
vars = c("n_tot_events", "n_events", "median", "hr", "ci", "pval"),
time_unit = adtte_f$AVALU[1]
)
#> B: Placebo A: Drug X
#> Baseline Risk Factors Total Events Events Median (DAYS) Events Median (DAYS) Hazard Ratio 95% Wald CI p-value (log-rank)
#> ————————————————————————————————————————————————————————————————————————————————————————————————————————————————————————————————————————————————
#> Total Patients 101 57 727.8 44 974.6 0.71 (0.48, 1.06) 0.0905
#> Sex
#> F 55 31 599.2 24 1016.3 0.56 (0.32, 0.96) 0.0341
#> M 46 26 888.5 20 974.6 0.91 (0.50, 1.65) 0.7558
#> Continuous Level Biomarker 2
#> LOW 36 21 735.5 15 974.6 0.76 (0.39, 1.51) 0.4324
#> MEDIUM 31 14 731.8 17 964.2 0.77 (0.36, 1.61) 0.4786
#> HIGH 34 22 654.8 12 1016.3 0.67 (0.33, 1.36) 0.2629